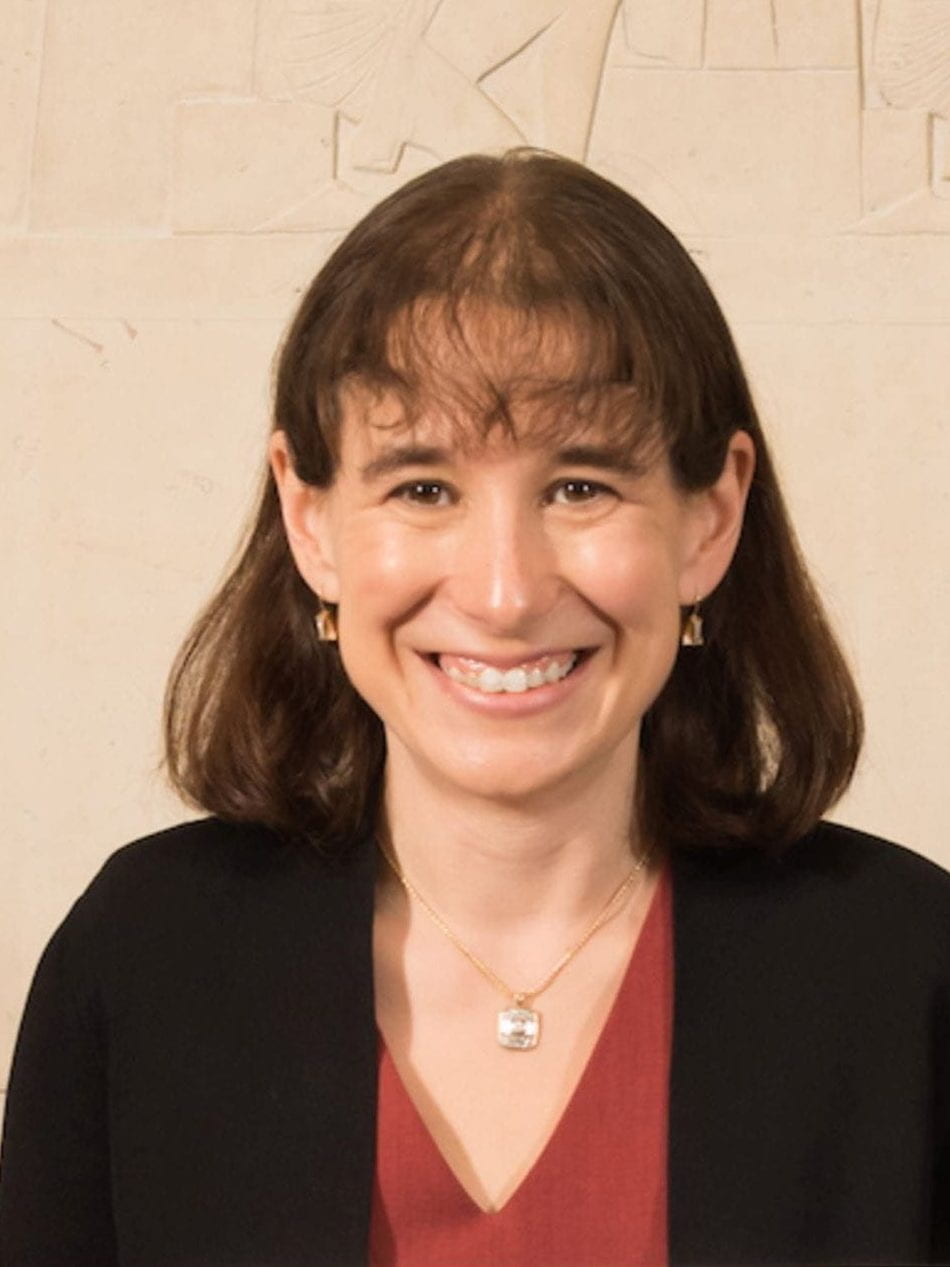
Marlene Cohen, Ph.D.
University of Chicago
“A many-tooled approach to studying the neural basis of flexible behavior”
Abstract
For good reason, most systems and computational studies focus on understanding the brain mechanisms behind a simple cognitive, perceptual, or motor process. This reductionist approach to behavior makes a lot of sense because it makes the problem tractable, and we hope that what we learn from simple situations generalizes to more complex situations. However, natural behaviors often involve many processes interacting at once. And while few disorders affect simple processes in isolation, the inability to flexibly adapt to the changing conditions that characterize real world behavior is a debilitating symptom of disorders ranging from autism to dementia to substance abuse. The complexity of more naturalistic behaviors means that studying them will require bringing together multiple experimental, computational, and theoretical tools.
I will present our recent and ongoing work focused on the neural basis of perceptual decision-making amid dynamically changing task demands. In natural environments, visually guided decision-making involves two indispensable processes: 1) identifying the relevant problem to solve (updating task-beliefs for appropriate task-switching), and 2) correctly solving the believed-relevant problem (perceptual decision-making). In laboratory studies, those two processes have been extensively studied separately, by requiring subjects to switch between very easy tasks or by studying perceptual decision-making under static task demands. We studied task-switching and perceptual decision-making together by using a dynamic, two-feature discrimination task that requires subjects to infer the visual feature whose correct discrimination will be rewarded.
Despite the fact that task switching and perceptual decision-making are thought to depend largely on different brain areas, we demonstrated a competitive link between them: when subjects performed well on the perceptual task, they were slower to notice that the task had changed, and vice versa. To understand the neural reason for this behavioral link, we used a combination of techniques embraced by the CRCNS community: multi-neuron, multi-area recordings in behaving animals, causal manipulations, hypothesis-driven dimensionality reduction techniques, normative and recurrent network modeling, and human psychophysics. As our field shifts toward studying more complex and naturalistic behaviors, this work has implications for methodology, basic science, and clinical applications. Methodologically, this work highlights the value of deeply integrating experimental and computational approaches. Scientifically, we demonstrated inextricable links between two disparate processes, highlighting the need to move (cautiously) away from a reductionist behavioral approach. And clinically, the neuronal link between perception and task switching suggests that potential treatments of disorders that affect decision-making under dynamic conditions might do well to target neurotransmitters and other processes that mediate communication between brain areas.
Biosketch
Marlene Cohen is a professor in the Department of Neurobiology at the University of Chicago. She received bachelors’ degrees in mathematics and in brain and cognitive science from the Massachusetts Institute of Technology. She received her Ph.D. from Stanford University working with Bill Newsome, studying how interactions between neurons depend on how animals plan to use the sensory information they encode. Her postdoctoral research with John Maunsell at Harvard Medical School used visual attention as a tool to understand which aspects of a cortical population code are important. She was a member of the faculty at the University of Pittsburgh from 2011 until her lab moved to the University of Chicago in June 2022. Her group uses a deep integration of physiological, behavioral and computational methods to study how visual information is encoded in the visual cortex, what information the visual cortex transmits to downstream areas, and the relationship between cognition, perception, and behavior. She has received awards including the Eppendorf and Science Prize for Neurobiology, a Klingenstein-Simons Fellowship Award in the Neurosciences, a Whitehall Foundation Grant, an Alfred P. Sloan Research Fellowship, and the Troland Research Award from the National Academy of Sciences. She works with many organizations aimed at improving diversity in science and beyond.
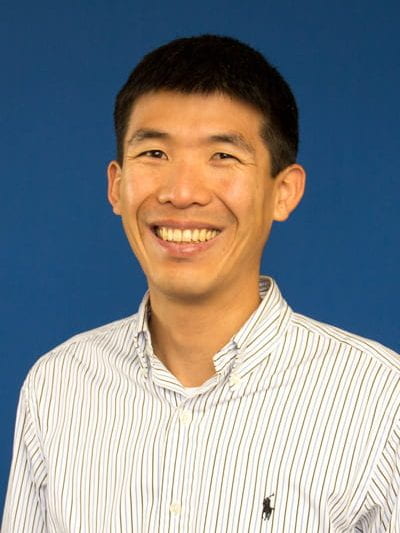
Byron Yu, Ph.D.
Carnegie Mellon University
“Brain-computer interfaces for basic science”
Abstract
Brain-computer interfaces (BCI) translate neural activity into movements of a computer cursor or robotic limb. BCIs are known for their ability to assist paralyzed patients. A lesser known, but
increasingly important, use of BCIs is their ability to further our basic scientific understanding of brain function. In particular, BCIs are providing insights into the neural mechanisms underlying
sensorimotor control that are currently difficult to obtain using limb movements. In this talk, I will demonstrate how a BCI can be leveraged to study how the brain learns. Specifically, I will address
why learning some tasks is easier than others, as well as how populations of neurons change their activity in concert during learning.
Biosketch
Byron Yu is the Gerard G. Elia Career Development Professor in Electrical & Computer Engineering and Biomedical Engineering at Carnegie Mellon University. He received the B.S. degree in Electrical
Engineering and Computer Sciences from the University of California, Berkeley, and the M.S. and Ph.D. degrees in Electrical Engineering from Stanford University. He was a postdoctoral fellow jointly in Electrical Engineering and Neuroscience at Stanford University and at the Gatsby Computational Neuroscience Unit, University College London. He is broadly interested in how large populations of neurons process
information, from encoding sensory stimuli to driving motor actions. His group develops and applies novel statistical algorithms and uses
brain-computer interfaces to study brain function.